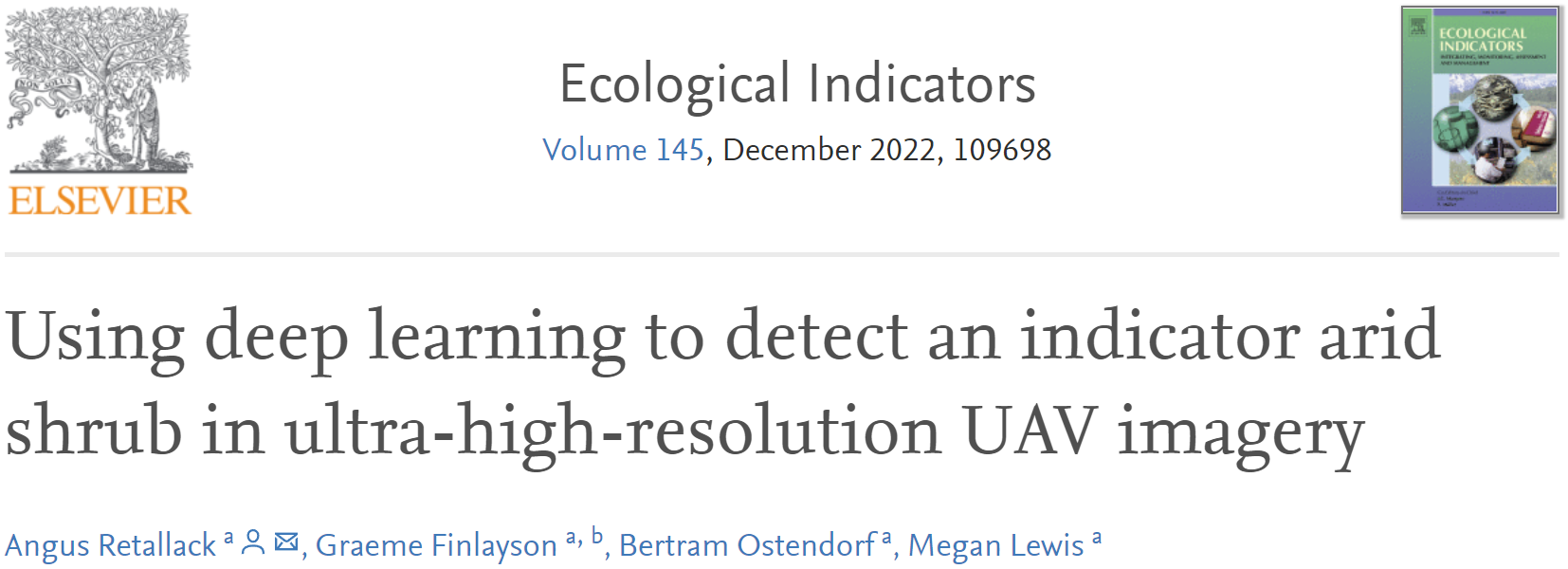
Article link: Using deep learning to detect an indicator arid shrub in ultra-high-resolution UAV imagery
My PhD research is in collaboration with Bush Heritage Australia. The second chapter of my thesis concerns the use of UAV imagery for gathering species-composition information, a component of biodiversity where measurement has typically been restricted to on-ground methods.
I used high resolution UAV imagery and deep-learning-based object detection models to automatically detect pearl bluebush (Maireana sedifolia) in UAV orthomosaics. The aim of the research was to assess the feasibility of this approach for use in monitoring programmes, as well as how detection accuracies were affected by the deep learning models chosen, image resolution, and monitoring site.
Overall, methods were able to achieve accuracies (F1 scores) centered around 75 – 80 %, with models detecting bluebush with accuracies greater than 90% at certain monitoring sites.
Bluebush detected by deep learning model
Importantly for potential implementation into ecological monitoring, there was no decrease in accuracy when using coarser resolution imagery (between 0.8 cm, 2 cm & 3 cm). This is significant due to the reduced processing times and greater imaging area able to be achieved by flying higher above ground level. Flying at ~ 110 m (3 cm resolution) enables around 16 x greater area to be imaged in the same period of time compared to flying at 30 m (~0.8 cm).
Additionally, detection accuracy was not significantly different when using a ResNet CNN with 50 or more layers. Therefore, ResNet-50 can be used (as opposed to ResNet-101 or 152), saving processing time with no loss in detection accuracy.
Therefore, results suggest that using the Faster R-CNN object detector with ResNet-50 and 3 cm UAV imagery is the most effective method for detecting pearl bluebush.
Read the full article at the link below.
Using deep learning to detect an indicator arid shrub in ultra-high-resolution UAV imagery
Leave a Reply